Do More with Twitter Data | How to Provide Customers with Unique Insights Faster
By: Datastreamer Team
April 2023 | 10 min. read
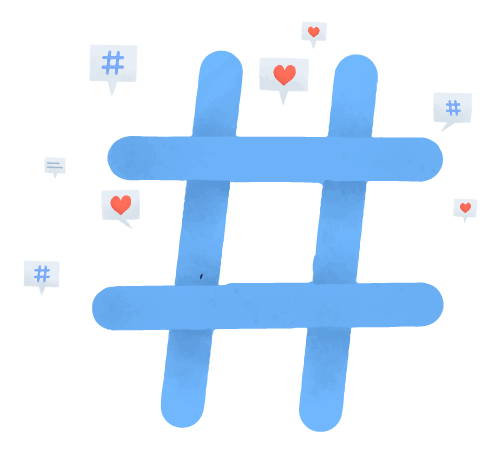
Twitter Enterprise API Changes
The recent changes to Twitter API’s has enterprises scrambling to adapt. If your organization offers an analytics product that utilizes third-hand social media data, the pricing adjustments might be alarming for your bottom line. Teams that consume high volumes of PowerTrack or Search have had to step back and evaluate the value vs. cost of these endpoints.
Your customers still expect decision-driving information to be delivered, fast. It’s already a daunting task to get quantitative recommendations from qualitative perspectives buried under petabytes of text data. Fortunately, there are tools that save your analysts hours of work while getting competitively unique information for threat detection, consumer insights, or research intelligence.
Protect Revenue by Extending Value
We’ve seen an influx of inquiries from enterprises that are looking for ways to either:
- Reduce the costs of Twitter data ingestion & transformation
- Increase the value of the analytics you provide to customers by unlocking rich insights that were previously inaccessible
We help organizations do both, but this article focuses on the latter – we list machine learning models that extend the analytical capabilities of your team while saving time. We also mention methods to streamline your data infrastructure to reduce operating costs.
Adding Power to PowerTrack & Search
The PowerTrack and Search API’s are powerful tools provided by Twitter. However, keyword searches and generalized tools often miss important information. Understanding factors like sentiment or intent can be impractical using keyword searches alone.
Datastreamer helps you run models that enrich data with the granular details you need for greater analytical confidence, without the need for specialized IT personnel to maintain data pipelines in-house. Plug in third-party data from Twitter or other vendors effortlessly through a simple interface. In addition to data enrichment you can combine Twitter with other data sources, run searches, and get real-time coverage all within a single platform.
Streamlining Twitter Data Pipelines
Although the price to access Twitter data is increasing, you can reduce operating costs indirectly by streamlining the infrastructure that feeds data into your product. For example, using a pre-built data pipeline that specializes in handling third party social media data can eliminate 95% of the labor that comes with transforming unstructured text data into an analytics-ready format.
Companies such as iThreat (Threat Detection) and LinkAlong (Research Insights) have seen these results after implementing a pre-built data pipeline:
- Reduced time analysts spend on research by 12x
- A single pipeline to feed 3 Billion+ documents per month (social media, news, blogs, forums) into their analytics product
- Cost savings (~$750K/year) by removing the need to develop and maintain infrastructure in-house
- Faster speed to market for a domain-specific AI model
- Improved speed, coverage, and accuracy of reports for clients
Machine Learning Models to Enhance Twitter Data
Location Inference Classifier for Twitter
The location inference classifier displays the city, region and country of origin of a media post. It can be used in conjunction with other classifiers and search terms to find & filter location-specific content.
Analysts can use this model to support the following use cases:
- Supplementing keywords in searches: Location inference can give a more city-level or country level view of content than relying on keywords or language.
- Removing specific areas from general queries: Location inference can be used in its inverse to remove certain cities from the results of content in a specific area.
In conjunction with aggregations and sentiment, high-level assessments of sentiment towards a brand in a specific city or country could be delivered to a product’s dashboard.
Violence Classifier for Twitter
The purpose of the violence classifier is to help identify instances of violence and threats as they occur on social media like Twitter, without reliance on extensive keyword lists that may or may not occur in the content.
Analysts can use this model to get faster answers to questions like:
- Is there any violent content associated with this person or entity?
- What is the rate or violence potential at a particular location?
Compared to a regular keyword search, the primary benefit of this model is to reduce false hits that may arise from the use of violent related keywords. For example, the statement “that movie was the bomb” would be classified as non-threatening due to the nonstandard use of the word “bomb”, whereas “there is a bomb at the airport” would be classified as violent. A keyword search does not make this distinction.
Intent Classifier for Twitter
The purpose of the intent classification model is to understand what intentions and human goals are referenced in a given media post.
Analysts can use this model to get faster answers to questions like:
- Are potential customers planning on buying a particular product?
- What kinds of customer behavior is associated with a particular market or location?
- Are there certain periods of time where there is increased interest in a particular product or company?
Extracting intentions from user generated content provides valuable insights. However, given the very large amount of this content, extracting intentions through the use of keywords is impractical, time consuming and expensive.
The Intent Classification model from Datastreamer allows a user to extract posts about planned future actions as a binary yes or no value from streams of Twitter data. It is a general intent classifier, meaning that it can encompass behavior such as purchasing, inquiring, criticizing, comparing, visiting, and selling.
Sentiment Classifier for Twitter
The purpose of the sentiment classifier is to take text posts in twitter and analyze if they are in general positive, negative, or neutral.
Analysts can use this model to get faster answers to questions like:
- Are people posting positive or negative things about my company or product?
- Are there periods of time when a product is looked at favorably on social media, for example, after a marketing campaign?
Rather than having analysts look for this information through the use of keywords such as ‘great’, ‘awful’, or ‘not too bad’, the sentiment classifier makes an assessment of sentiment based on generalized learned patterns in language.
Feed Specialized AI Models with Twitter Data
If your company offers insights for domain-specific information, you might run into limitations using general platforms and tools. Typically you’ll have to find or build a custom AI model with domain-specific taxonomies. Feeding Twitter data into specialized models improves outcome accuracy by better filtering queries with relevant information.
For example, our customer LinkAlong, built an AI-driven product to support researchers at the World Health Organization and the International Red Cross. LinkAlong’s domain specific solution helped analysts formulate questions easily and find precise answers from large amounts of social media, news, blogs, and forum data. Their AI product ultimately led to analysts reducing the time spent on research by 12x.
💡LinkAlong & Datastreamer
LinkAlong used Datastreamer to build the pipeline that fed their AI product with 3+ billion documents monthly. Our solution delivered a full streaming API that handles 95% of data indexing requirements. This removed the roadblock LinkAlong had to run powerful queries and aggregations with raw data.
Combine Twitter Data with Multiple Sources In A Single Platform
To get the “full picture” of consumer perception or threat risk, you need to be able to analyze user posts from multiple sources. Being able to find common threads scattered on different channels can help you spot relevant information faster.
But data teams are familiar with the time-intensive work that goes into transforming unstructured user-generated text into a useful machine readable format. This challenge amplifies remarkably when you want to work with multiple external data sets simultaneously.
You need to worry about standardizing schema, indexing data, and creating real-time streams, all of which can take months to develop internally. Even if you decide to purchase a data pipeline, there are very few vendors that work with unstructured external data all.
Datastreamer is one of them. Adding new data sources into your pipeline takes seconds. You can combine, filter, aggregate, and run real time enrichments on data from various sources through simple parameter changes.
💡 Our average customer ingests 8+ unique data sources concurrently
Our partnerships with world-class data providers enable you to access billions of pieces of unstructured, high-quality data from millions of sources. Supplement Twitter data with other social media, news, blogs, forums, dark web data, and more.
Adapt Faster with A Managed Infrastructure
Managing data pipelines in-house can be a nightmare. Every time Twitter or other data sources make a change to their API, your technical personnel need to be quick to respond. This can drain time away from other efforts which slows down your roadmap.
You can eliminate this problem by plugging in to a managed infrastructure that was specifically built to handle large volumes of unstructured text data. Our data scientists and developers not only keep data pipelines up to date with market changes but they are proactively adding features that push the technical boundaries of NLP on massive data sets, which give you a competitive edge.
Focus on your product while our existing infrastructure and experts take care of the engineering challenges that come with scaling the ingestion, transformation, and enrichment of massive external data sets.
About Datastreamer
Datastreamer is a turnkey data pipeline platform. Our solution is the layer between data suppliers and data consumers that removes 95% of the work required to transform unstructured data from multiple external sources into a unified analytics-ready format. Source, combine, and enrich data effortlessly through a simple API interface to save months of development time. Plug existing components from your pipelines into our managed infrastructure to scale with less overhead costs.
Customers use Datastreamer to feed text data into AI models and power insights for Threat Intelligence, KYC/AML, Consumer Insights, Financial Analysis, and more.